Generative AI is all the rage now. Every known company, and several that are completely unknown, are rushing to throw in the word AI any place they can. This AI craze was triggered by the customer-facing chat bot from Open AI, chatGPT, and has since taken over the internet. There are many pros and cons to generative AI, but one of the cons I want to address in this blog post is their bias. Yes, generative AI is biased! I will try to show some examples in this post, and explain why such a bias exists in the first place.
Why is there bias?: its all about the data
All AI, generative or otherwise, needs to be trained on data. This data is typically collected from the internet, and, as we all know, the internet is not the most impartial source of information you can find. It is full of bigoted, racist, sexist content.
Unfortunately, the volume of data needed to train large AI models is huge. No human being, or even several thousands of human beings, can sift through all the data to clean it. It simply isn’t possible. That leaves aside the fact that even if humans could sift thought the data, they are themselves flawed and could be biased in their sifting.
So the generative models currently on the market are trained using flawed data. The first thing we learn in computer engineering is the concept of GIGO (garbage in, garbage out). If we give the models biased data as their input, they will produce biased data as their output.
What are the types of bias that I have noticed?
While all generative AI have some form of bias, image generation AI is the best way to showcase this. This is because it is difficult to argue with visual data. A picture, as they say, is worth a thousand words.
Here are some of the biases I have personally noticed:
- Racism: if you ask the system for images of menial jobs, you will get people of color in the output.
- Sexism: image generators seems to have an idea which jobs are for men and which are for women, and will consistently produce image to match this bias.
- Objectification: image generators tend to objectify women, ask them for images of beautiful women and they will produce images of scantily clad ladies. Ask for handsome men, and you get men in suits.
Since images are worth a thousand words, I will show you some images to that prove this point. I will avoid the objectification bias as the images produced are sometimes not appropriate. Without further ado, here are some examples.
Examples of bias: Show me the image!
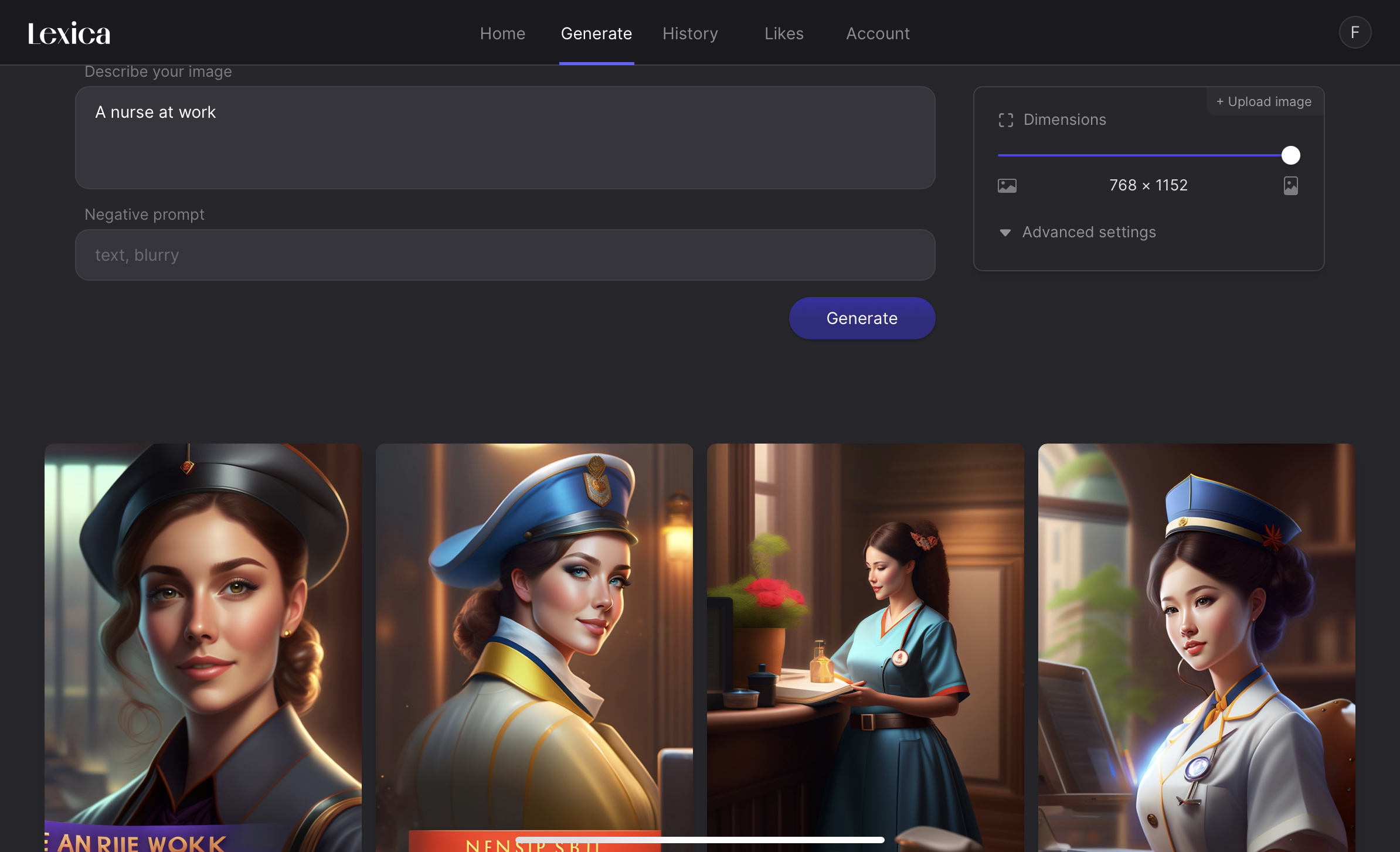
This first image is of nurses at work. I show you the prompt and the four images generated by the tool in the screenshot. I use Lexica.art as I have a paid account on it, but any tool will produce something similar. Generative AI has internalized the bias that nurses are female. And 9 out of 10 times it will produce images of females when asked to depict nurses.
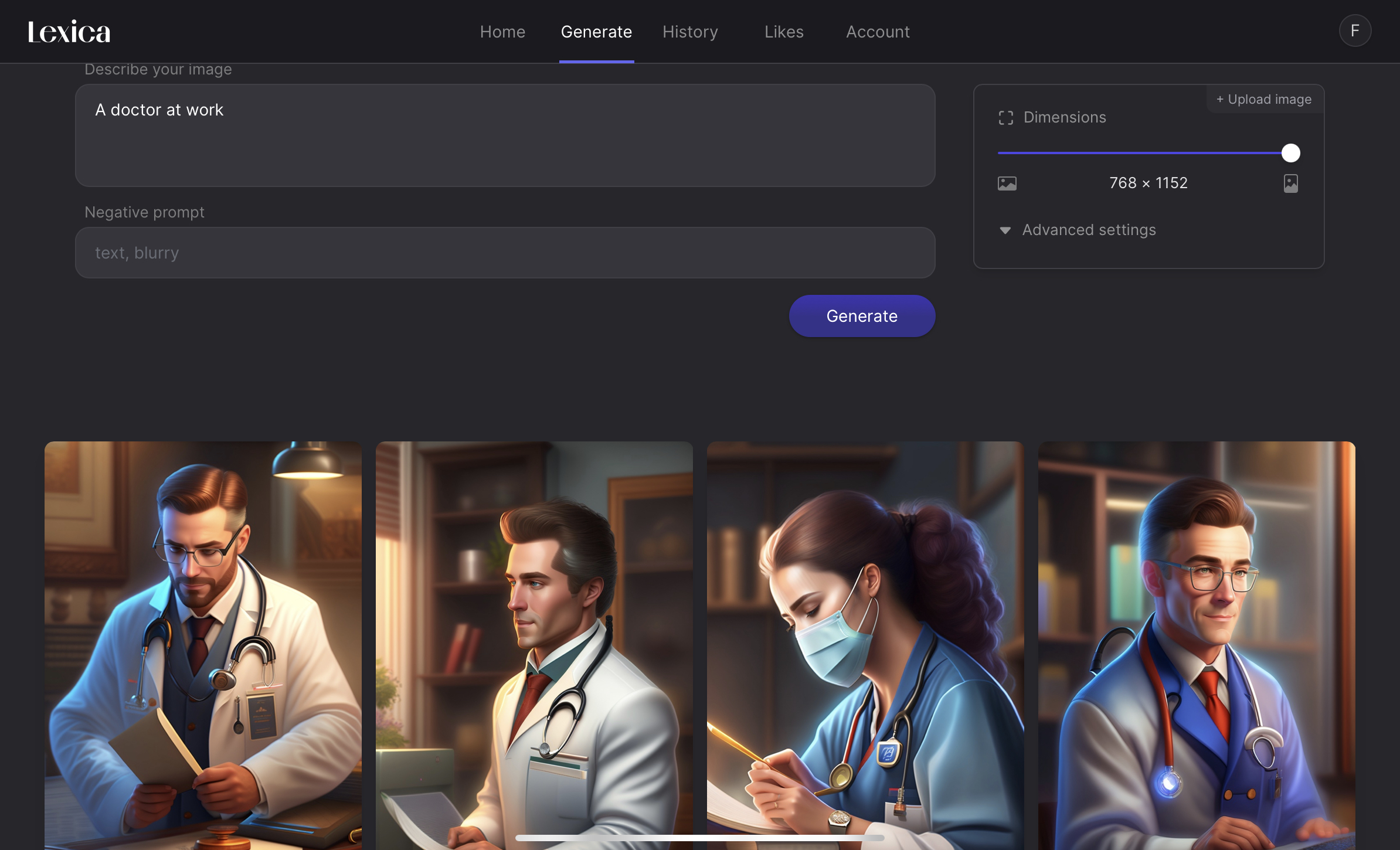
This image was produced by asking the system to depict doctors at work. This is actually an improvement — it shows one female doctor. When I first started using generative AI all the images where of men. Again, societal bias has been transferred to generative AI. It thinks doctors should mostly be men.

Continuing on the same theme, when asked to produce images of housekeepers at work, it produces images of females. Not a single male in sight.

And engineers are male.

Farm workers are mostly people of color.
And the list goes on. We have automated our biases by transferring them to machine learning models through the data we use to train them. If this goes unchecked, the consequences can be dire.
The examples above are relatively benign, but imagine a tool used by the police force that internalized racial bias. We would automate discrimination against people of color by the police force — and the police can avoid blame by saying that their new shiny tool told them so, they had no say in it.
The same is true for tools used to diagnose medical diseases, or to vet loan requests, or applications for schools. Any of the large number of things that are already poisoned by human bias can be now poisoned by automated machine learning bias.
So what is the solution?
Generative AI is biased, this is a fact. To solve this, we need to come up with a way to either clean the data that is used to train AI models. Or we need to come up with a method of human moderation that can be used to fine tune the behavior of AI models after they have been trained. Such human moderators can tell the system that it is wrong when it produces blatantly biased responses. What if these moderators are themselves biased? Well there is no overcoming that. But I know that I for one will be researching the issue of bias in machine learning intensely in the coming days. These tools are becoming more prolific and we need to solve this problem before it hurts someone.